Any backtests or machine-learning models based on standard datasets will be inaccurate. This is because the figures available today are different to those available in the past.
Most backtests are currently run against standard datasets, whether fundamental or economic, that do not tell the full story behind the figures. These datasets include the latest reported values for any datapoint, but do not show that those values may have been revised and restated several times, for example due to divestitures, mergers and acquisitions or accounting changes. These revisions are common across all industries and can be made weeks, months or even years after the original filing, to significant effect.
In standard datasets, these restated figures will overwrite historical results, meaning that your backtest can only take into account the current value and not the information that was actually available at the time. This has the potential to seriously impact the quality of your research, and the reliability of your results.
What is portfolio backtesting?
Portfolio backtesting is the process of simulating an investment strategy using historical prices to test how well the strategy would have done in the past. Running a simulation over a large number of stocks for past decades is a computationally intensive process. But if you are testing and building models with standard datasets, you are using inaccurate data that may render the results of those tests less reliable – jeopardizing your entire process.
Look ahead bias occurs when research or a simulation relies on data or information that was not yet available or known during the time period being studied. You may be applying lag assumptions to mitigate these risks – however these lags are also imprecise. Complicated by filings regulations that change over time and differ between countries and companies, they are often an ineffective solution to the problem.
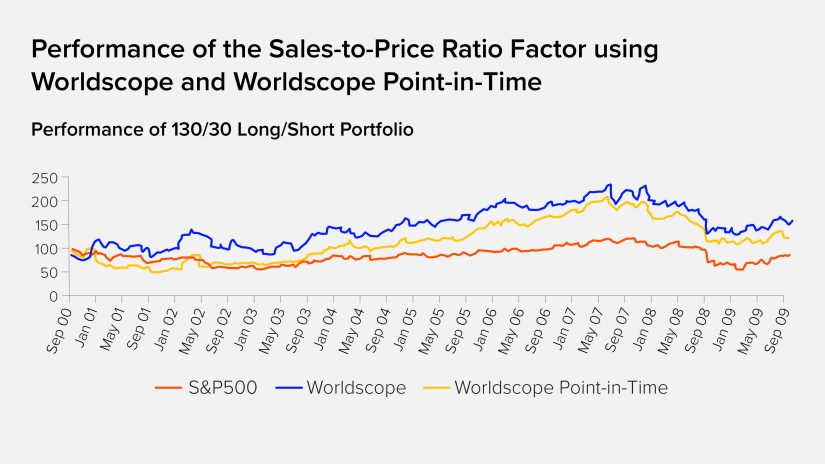
Our solution
Transform the way you backtest quantitative strategies
As the only dataset to include all reported values, timestamped to the moment that they were made available to the market, LSEG Point-in-Time data is unique in showing you exactly what information was available and when. This allows you to backtest against real conditions – removing the need for inaccurate lag assumptions and allowing for more accurate assignment to groupings. This ultimately provides more accurate results, and a truer analysis of how your portfolio or strategy would have performed.
With two Point-in-Time fundamentals databases, and the market’s only Point-in-Time estimates data, we offer the industry’s most comprehensive offering of Point-in-Time data. Every datapoint is regularly updated and has the high standard of accuracy you expect from LSEG.
Point In time data | Non Point In Time data |
Has all figures, including preliminary results, finalized results and restatements.
|
Includes only the most recently available figures, overwriting previous results. |
Timestamped to the exact date and time the information was made available. | A lag period must be assumed relative to the filing period end date. |
Includes data on funds and entities that existed at the time. | Includes data only on funds and entities that exist today. |
What our data covers
- LSEG Worldscope Fundamentals: Coverage of almost 90,000 companies, dating back to 1983, timestamped to the day and updated daily.
- LSEG Financials: Coverage of over 100,000 companies, dating back to 1980, timestamped to the day and updated daily.
- LSEG I/B/E/S: The only Point-in-Time Estimates data available in the industry. Coverage of over 80,000 companies, dating back to 1980, updated weekly with daily timestamping. Economics Point-in-Time Over 2,100 key indicator economic series, with up to 20 years of history.
- Economics: Coverage of 52 countries, dating back to 2010, time stamped to the day and updated on an intraday basis
- Reuters Real-time News: Coverage of over 90,000 companies, dating back to 1996, timestamped to the millisecond and updated in real-time.
- LSEG MarketPsych ESG Analytics: More than 20,000 active companies from over 80 countries, with history dating back to 1998.
- The LSEG Business Classifications: Covering over 250,000 securities in 130 countries to 5 levels of granularity, The LSEG Business Classifications (TRBC) is the most comprehensive, detailed, and up-to-date sector and industry classification available
- LSEG MarketPsych Analytics: coverage of 16,000+ companies, 36 commodities and energy subjects, 187 countries, 62 sovereign markets, 45 currencies, 150+ cryptocurrencies, dating back to 2011
Benefits
Begin portfolio backtesting with confidence
Biases are inherent in standard data, point-in-time data helps eliminate survivorship and look-ahead bias.
Use historic data that is consistent and never overwritten to ensure the results of your models are accurate.
Datasets include preliminary, original, re-statements, reclassifications, and final figures, as far back as 1980.
If we are not using point-in-time data, we could end up with a backtest that is not properly representative of a strategy’s historical performance. Any subsequent trading decision based on such a backtest could be made as a result of inaccurate data. We could end up investing in a strategy that is not as attractive as our statistical analysis suggests, because of hindsight bias induced by using data which isn’t point-in-time.
Saeed Amen
Request details
Call your local sales team
Americas
All countries (toll free): +1 800 427 7570
Brazil: +55 11 47009629
Argentina: +54 11 53546700
Chile: +56 2 24838932
Mexico: +52 55 80005740
Colombia: +57 1 4419404
Europe, Middle East, Africa
Europe: +442045302020
Africa: +27 11 775 3188
Middle East & North Africa: 800035704182
Asia Pacific (Sub-Regional)
Australia & Pacific Islands: +612 8066 2494
China mainland: +86 10 6627 1095
Hong Kong & Macau: +852 3077 5499
India, Bangladesh, Nepal, Maldives & Sri Lanka:
+91 22 6180 7525
Indonesia: +622150960350
Japan: +813 6743 6515
Korea: +822 3478 4303
Malaysia & Brunei: +603 7 724 0502
New Zealand: +64 9913 6203
Philippines: 180 089 094 050 (Globe) or
180 014 410 639 (PLDT)
Singapore and all non-listed ASEAN Countries:
+65 6415 5484
Taiwan: +886 2 7734 4677
Thailand & Laos: +662 844 9576